tf.keras.layers.LSTM 의 속성 return_sequences를 True 로 설정하면 각 시퀀스별 은닉 상태(hidden state)를 모두 출력하게 된다.
다음 그림은 시퀀스 길이가 4
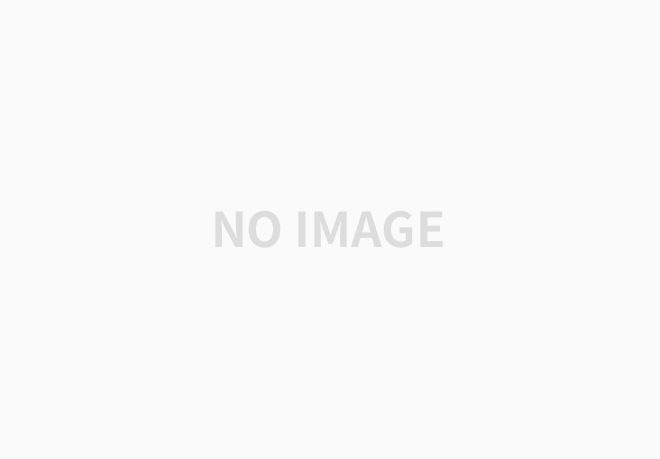
이 모델의 특징은 각 시퀀스에서 은닉 상태가 모두 출력된다는 것이다. 이를 코드로 구현하면 다음과 같다.
input = tf.keras.layers.Input(shape=(4, 8)) # (seq length, input dimension)
lstm = tf.keras.layers.LSTM(units=3, return_sequences=True)
out = lstm(input)
print(out.shape)
출력의 사이즈는 (4,3) 이 나온다. 4개 시퀀스에서 차원이 3인 은닉 상태가 출력됐기 때문이다. 반면 return_sequences=False로 하면 출력 사이즈가 3이 된다. 마지막 시뭔스에서만 은닉 상태가 출력되기 때문이다. 디폴트는 return_sequences=False 이다.
return_state = True 를 한 경우에는 마지막 시퀀스에서의 출력(은닉 상태), 은닉 상태와 셀 상태 값이 출력된다. 출력은 은닉 상태와 동일하다.
input = tf.keras.layers.Input(shape=(4, 8)) # (seq length, input dimension)
lstm = tf.keras.layers.LSTM(units=3, return_state=True)
out, h_st, c_st = lstm(input)
print(out.shape, h_st.shape, c_st.shape)
코드에서 out는 출력, h_st는 은닉 상태, c_st는 셀 상태이며 각각의 사이즈는 3, 3, 3 이다.
return_sequences와 return_state를 모두 True로 하게 되면 각 시퀀스별 은닉 상태와 마지막 은닉 상태, 마지막 셀 상태 값이 출력된다.
input = tf.keras.layers.Input(shape=(4, 8)) # (seq length, input dimension)
lstm = tf.keras.layers.LSTM(units=3, return_sequences=True, return_state=True)
out, h_st, c_st = lstm(input)
print(out.shape, h_st.shape, c_st.shape)
코드에서 out는 출력으로서 각 시퀀스 별 은닉 상태, h_st는 마지막 은닉 상태, c_st는 마지막 셀 상태이며 각각의 사이즈는 (4, 3), 3, 3 이다.
LSTM 을 다층 구조로 만들 수도 있다. LSTM 위에 다른 LSTM을 쌓아 올리는 구조로서 multi-layered LSTM 또는 stacked LSTM 이라고 한다. 다층 LSTM에서는 아래 층의 모든 시퀀스의 출력이 위 층의 시퀀스로 전달되어야 하기 때문에 return_sequences=True 를 사용해야 한다.
다음 그림은 2층으로 된 LSTM을 나타낸다. 시퀀스 길이가 4
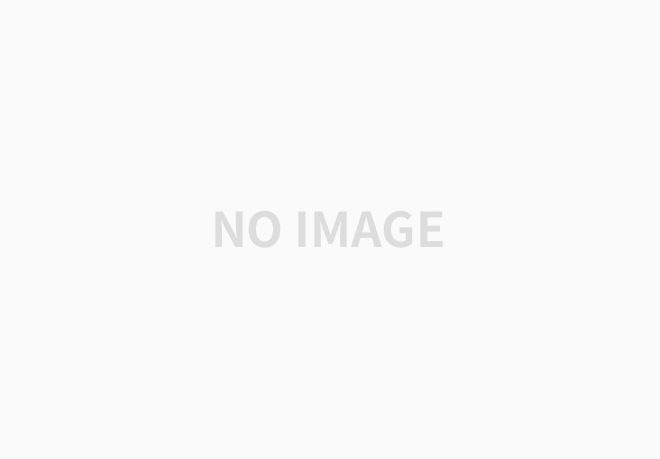
이 모델을 코드로 구현하면 다음과 같다.
input = tf.keras.layers.Input(shape=(4, 8)) # (seq length, input dimension)
lstm_1 = tf.keras.layers.LSTM(units=3, return_sequences=True)(input)
lstm_2 = tf.keras.layers.LSTM(units=5)(lstm_1)
model = tf.keras.models.Model(inputs=input, outputs=lstm_2)
model.summary()
Model: "model"
_________________________________________________________________
Layer (type) Output Shape Param #
=================================================================
input_1 (InputLayer) [(None, 4, 8)] 0
lstm (LSTM) (None, 4, 3) 144
lstm_1 (LSTM) (None, 5) 180
=================================================================
Total params: 324
Trainable params: 324
Non-trainable params: 0
1층은 모든 시퀀스에서 은닉 상태를 출력해야 하기 때문에 return_sequences=True 를 사용했지만, 2층은 마지막 시퀀스에서만 출력이 필요하기 때문에 이 속성을 사용하지 않았다. 따라서 1층의 출력 사이즈는 (4, 3)이, 2층의 출력 사이즈는 5 가 나온다.
'AI 딥러닝 > Sequence' 카테고리의 다른 글
[seq2seq] 어텐션이 포함된 seq2seq 모델 (0) | 2023.08.23 |
---|---|
[seq2seq] 간단한 seq2seq 모델 구현 (0) | 2023.08.17 |
[LSTM] LSTM-AE를 이용한 시퀀스 데이터 이상 탐지 (0) | 2023.05.31 |
[LSTM] 주가 예측 (0) | 2023.05.19 |
[LSTM] TF2에서 단방향 LSTM 모델 구현 - 1 (0) | 2022.07.21 |
댓글