경사하강법(gradient descent)이 어떤 함수의 최소값을 향한 방향을 계산하는데 1차 미분을 사용하는 반면 뉴턴방법(Newton's method)는 2차 미분을 사용한다. 따라서 뉴턴방법이 경사하강법보다는 성능이 훨씬 좋다.
제약조건이 없는 최적화 문제는 다음과 같다.
여기서
뉴턴방법의 기본 개념은 최적화 변수의 시작값(starting point)
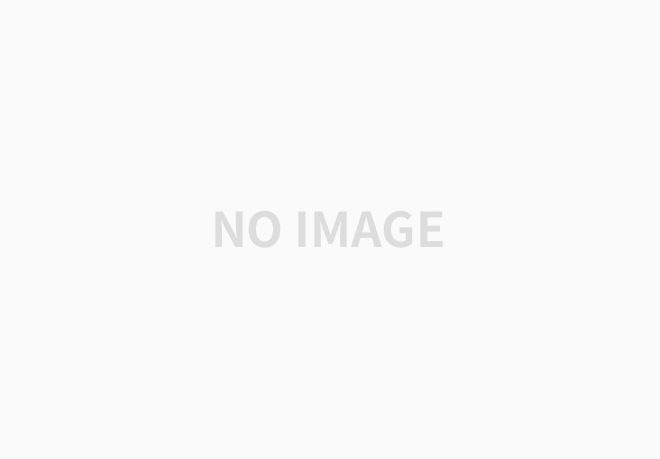
목적함수
여기서
따라서
여기서
뉴턴스텝
먼저
식 (7)에 의하면 결국
그렇다면 뉴턴방법으로 이터레이션하면서 구한
여기서
뉴턴감소량이 일정 수준 이하로 작아지면 최적해에 거의 근접했다는 신호로 볼 수 있으므로 이테레이션을 멈출 수 있다.
뉴턴방법을 정리하면 다음과 같다.
0. 시작값
1. 다음을 반복한다.
[1] 뉴턴스텝과 뉴턴감소량을 계산한다.
[2] 뉴턴감소량을 종료조건 값
[3] 스텝사이즈
[4]
뉴턴방법의 단점은 최적화 변수의 개수가 클 때 헤시안 행렬
'AI 수학 > 최적화' 카테고리의 다른 글
장벽 내부점 방법 (Barrier Interior-Point Method) (0) | 2022.04.13 |
---|---|
등식 제약조건에서의 뉴턴방법 (Newton’s Method) (0) | 2022.04.10 |
내부점 방법 (Interior-Point Method)의 개념 (0) | 2022.04.06 |
프라이멀 문제와 듀얼 문제의 유도 (0) | 2022.04.04 |
역전파 (Backpropagation) 계산 (0) | 2021.03.31 |
댓글